Detection of grapevine trunk disease Esca by hyperspectral imaging
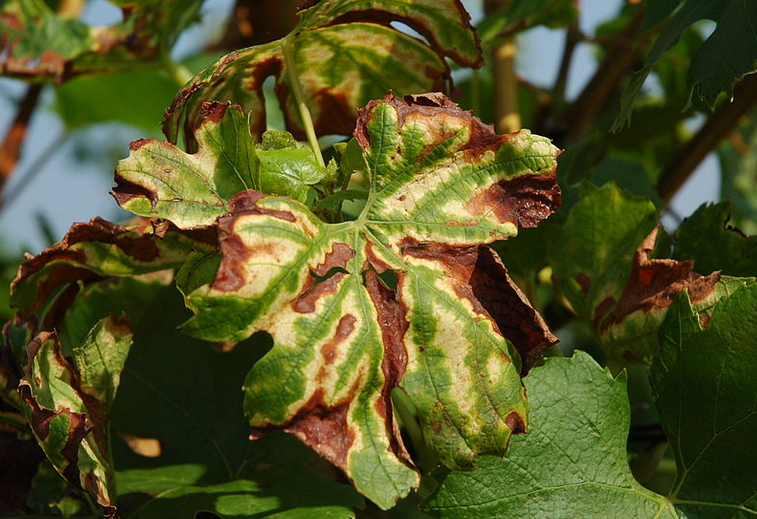
Global viticulture loses more than £1 billion every year due to so-called grapevine trunk diseases (GTD). Some vineyards just suffer a lower yield whereas others can lose the whole vineyard. Esca is one of three main GTD diseases. Unlike the usual seasonal problems such as downy or powdery mildew, GTD have no known treatment or cure. Sodium arsenite (NaAsO2) was used in the past to control GTD but due to its wide toxicity and accumulation in the environment, its agricultural use has been banned.
One of the perplexing features of Esca is that some leaves on a vine may be affected while others appear healthy; one year a vine may look very sick with highly discoloured leaves but the next year appear healthy. Usually it is a chronic disease but can cause sudden death in just a few days with little advanced warning.
Anna Kicherer and colleagues at the Institute for Grapevine Breeding in Siebeldingen, Germany have recently reported the use of hyperspectral leaf imaging to monitor Esca in a vineyard in Germany. Writing in the journal Plant Methods they compared aerial and land-based methods of recording the reflectivity of grapevine leaves. Spectral imaging techniques have the advantage that they are non-invasive, non-destructive and can characterise crops quickly. They give what medics call a presumptive diagnosis based on the appearance of disease rather than identifying a specific pathogen with a genetic test. Nonetheless this can be very valuable as spectral imaging is quantitative, objective and contains more information than visual inspection.
As Sir Isaac Newton said, the human eye is a wonderful invention but it sees only three colours: red, green and blue. Multispectral imaging has the advantage that it sees 5-7 different colour bands from the near ultraviolet (UV) to the near infrared (NIR). Hyperspectral imaging goes further and can comprise hundreds of different colour bands from the UV (400 nm wavelength light) to NIR (1000 nm wavelength light) and infrared (IR). The Siebeldingen group therefore made a comparison of visible-NIR and IR hyperspectral imaging in the vineyard and multispectral imaging from an Unmanned Aerial Vehicle (UAV) flown over the vineyard. Small, light weight multispectral cameras can be carried by drones but heavier hyperspectral cameras need to be used on the ground. They also modelled the potential optimisation of carefully chosen hyperspectral bands to measure Esca disease in vines.
To gather the hyperspectral images of vines in a local vineyard, Kicherer and team used a modified tractor equipped with imaging cameras and GPS. The figure below shows how it was deployed in the vineyard. The tractor was equipped with additional bright light sources and spectral cameras.
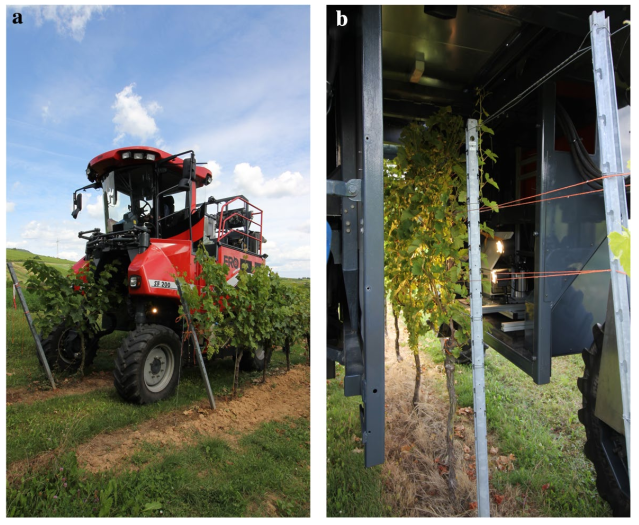
It was known at the outset that the vineyard had an incidence of around 11% Esca in the vines. Vines were imaged biweekly during the growing seasons over a three year period. Spectral imaging immediately revealed differences between healthy leaves and those affected by Esca as shown below.
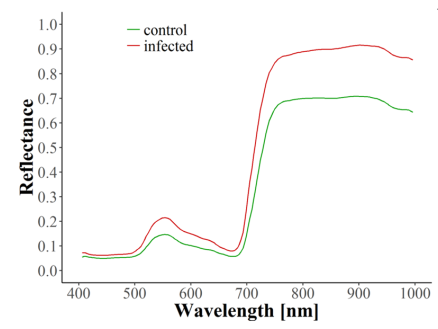
Infected leaves clearly reflect more NIR light (700-1000 nm) and yellow to red light (550-650 nm). Much smaller changes were seen in the IR wavelength range measurements. Taking measurements from two of the three years of study, it was possible to create a machine learning model to identify vine leaves showing Esca. By adding information about which leaves came from infected vines it was possible to improve the classification of the infected and healthy vines. In the best case, using visible-NIR hyperspectral data from two years and applying the model to the third year: classification was 82% accurate, the true positive rate was 85% and the false positive rate was 14%.
Encouraged by these results, Kicherer and team tested the classification model on pre-symptomatic Esca infected vines. They reported 73-81% classification accuracy, 69-100% true positive rate and 20-26% false positive rate. Finally they beat the human eye!
Perhaps in the future vineyards will be able to use spectral imaging to eradicate Esca infected vines before infection spreads to the rest of the plants.
The Plant Methods paper also contains further information on UAV measurements and ideas for future multispectral imaging approaches. To find out more, read the Open Access document here. Further information on Esca and other GTD is available in EU sponsored YouTube videos.
Figures are reproduced courtesy of a Collective Commons licence from Julius Kühn-Institut and BMC Springer Nature.
[ratemypost]