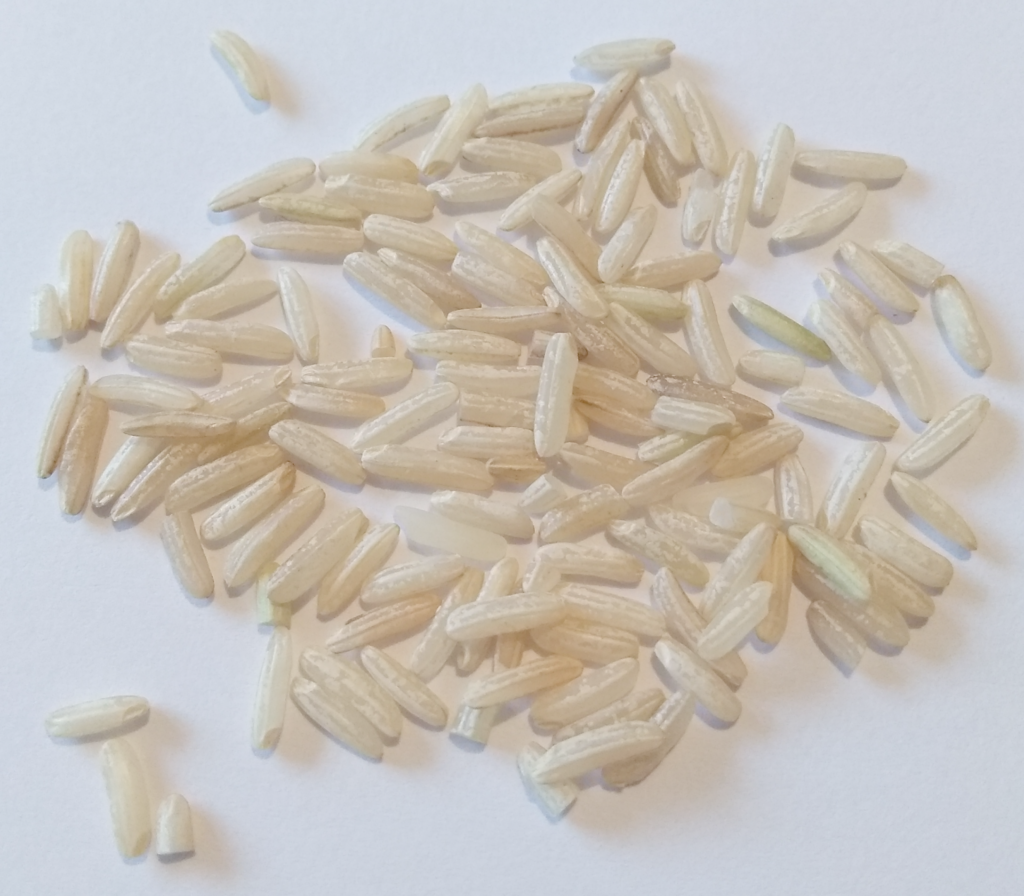
Hyperspectral imaging (HSI) sounds like something Superman should have. And indeed it is. Hyperspectral imaging is increasingly used in art conservation, waste management, industrial quality control, medicine and agriculture. HSI not only gives an image of an object like the smartphone photo above, it also contains a detailed spectrum of every single pixel in the image.
Most images you find on Google or Instagram are either black and white, greyscale or colour. Colour information is typically restricted to how much red, green and blue light make up a particular point. This is because cells in the human eye measure only these three colours. Spectral imaging, on the other hand, records many different wavelengths of light. Hyperspectral imaging records a wide range of wavelengths with high resolution.
Whipping out your smartphone and taking a picture of a map, a set of instructions or a business card has replaced note taking. Clicking an image is faster, more reliable and durable than laboriously writing on scraps of paper. You can return to a saved image and rather than having to de-cypher poor writing or gaps in instructions, you can zoom into more detail than you realised was there in the first place. The same is true of hyperspectral imaging compared to conventional measuring and documenting.
Researchers at the US Department of Agriculture (USDA) Dale Bumpers National Rice Research Center in Arkansas have used these advantages of hyperspectral imaging to demonstrate a quick and reliable way to assess rice quality. Rice is the second most important food staple globally and some 3 billion people rely on rice as their main foodstuff. It is a truly massive commodity and assessing rice quality quickly has global importance.
The USDA team’s research demonstrated HSI was capable of: distinguishing different types of rice; telling which soil type the rice was grown in; quantitatively measuring the chalkiness of a particular type of rice. This final capability is possibly the most valuable because so-called chalk in rice is due to agglomeration of starch particles within the seed and it makes the grains break when they are milled. Fractured grains are useless for human consumption and identifying batches of rice with a high chalk content prior to milling saves money and improves efficiency.

The methods used to uncover the hidden information in hyperspectral rice images are well-known to data scientists but less-so to agronomists. Dividing the spectra from the grain images into narrow wavelength windows, it was possible to find windows and combinations of window values that acted as components from which the spectrum of any pixel in the spectral image could be reconstructed. These Principle Components were then used to produce a calibration plot of HSI predicted %chalkiness (derived from Partial Least Squares/Discriminant Analysis PLS-DA) against true %chalkiness. The figure above shows a convincing linear relationship between the HSI parameter and the actual % chalk measured by SEM.
Hyperspectral rice images were also used to reveal which batches had been grown in Arkansas (AR09 & AR10) and which in Texas (TX08). Relative intensities of spectral windows are plotted in the figure below. PLS-DA analysis was used to make a classification of each of the three type of rice, TX08, AR09 and AR10. The red, green and blue rice batches can be clearly seen to cluster in distinct groups, with a small number of overlapping points.

Finally the hyperspectral rice images were used to demonstrate discrimination of three sub-species of rice: IND (indica), TRJ (tropical japonica) and TEJ (temperate japonica). The figure below shows again the differences in the spectral windows used in PLS-DA analysis of the three batches of rice.

The TRJ, TEJ and IND clusters are quite widely spread but clearly separated by analysis of the hyperspectral images.
A full account of the agronomy and the methods used in the study can be found at https://doi.org/10.1038/s41598-020-65999-7 in Nature Scientific Reports. Figures are used under Creative Commons with thanks to USDA and Nature. The full report also gives an interesting account of the genomics of the rice sub-species used and some consideration of Single Nucleotide Polymorphism (SNP) differences that may be seen in the hyperspectral phenotypes.