Smartphone spectral leaf imaging
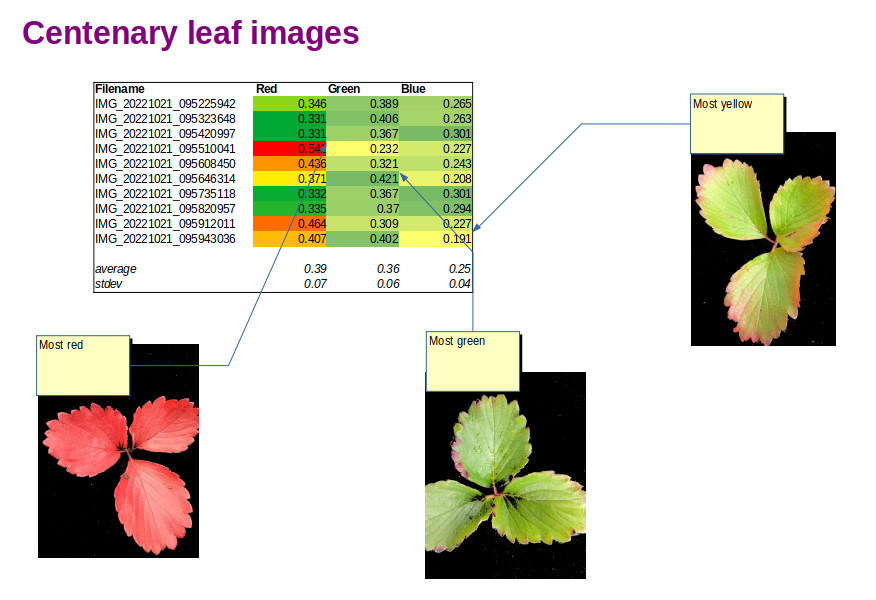
All season we have been monitoring the health of our Strawberry Greenhouse crop. In addition to visual inspection with a loupe, digital leaf imaging has been a useful way to follow the development of the plants. Now that autumn has arrived, the leaves are showing the usual spectacular colour changes.
Chlorophyll green quickly gives way to yellowing and eventual reddening of the leaves. The yellow colour pigments are usually present in the leaves but as chlorophyll production is much less stimulated by sunshine, the yellow pigments can be seen. Red pigments are increasingly produced in leaves as the sugar concentration in the leaves increases. Leaf yellows are due to carotenoid pigments and leaf red colours are due to anthocyanin pigments.
Chlorophyll molecules absorb both red and blue light, leaving green visible light to be reflected by plant leaves, making them appear green. Carotenoid molecules on the other hand absorb light in the blue end of the spectrum making leaves reflect and scatter yellow, green and red light. Anthocyanin molecules absorb blue and green light, making leaves reflect and scatter deeper red light.
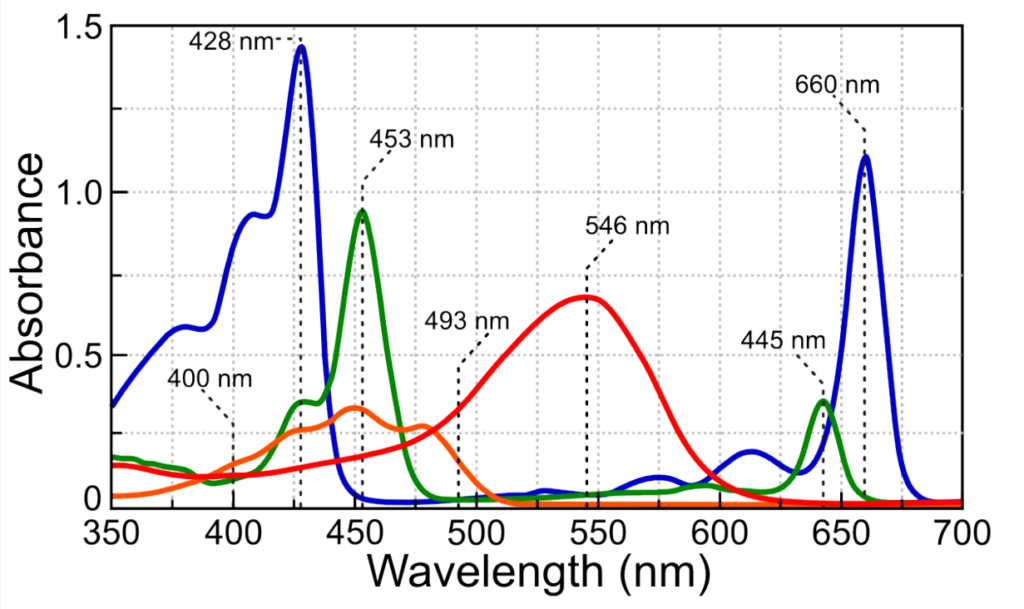
Spectrometers and hyperspectral imaging devices can measure the colours of light reflected by leaves with high precision (1 nm or better). As the figure above from researchers at the Universidad de Guadalajara in Mexico shows, the light absorbed by plant pigments is absorbed in wavelength bands much wider than 1nm. Modern smartphones typically have cameras with many megapixels, giving images of astonishing resolution. The detector chips also have RGB elements capable of measuring red, green and blue light. The different red, green and blue pixels do not have high spectral resolution, their sensitivity curves are rather broad. However the red, green and blue sensitivity curves are quite comparable to the widths of the absorption curves of the three main leaf pigments. The figure below shows typical spectral sensitivity curves for an Android phone.
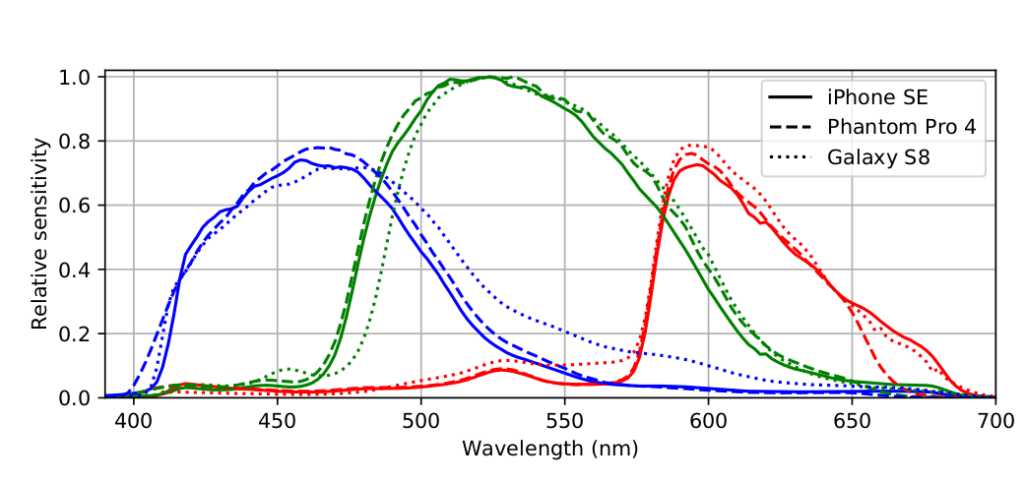
Red camera pixels preferentially detect light attenuated by chlorophyll and green pixels preferentially detect light attenuated by anthocyanins. Blue pixels are not so discriminating, they detect light attenuated by chlorophyll, carotenoids and to a lesser extent anthocyanins.
It should therefore be possible to construct an index or set of indices which relates at least qualitatively to the amounts of leaf pigments in strawberry plant leaves. However this is not straightforward, the red channel is the only colour which measures the influence of just one pigment, chlorophyll. Red channel values cannot be simply used directly because lighting intensity varies during the course of a day and the sky and sun colours also change subtly. Changes in illumination (colours and intensity) can be normalised to some extent using a reference background for leaf imaging. A uniform black coloured background provides a useful contrast to the leaves themselves as well as a reference for red, green and blue intensities measured by the smartphone camera.
The figure at the top of this blog shows a spreadsheet with three normalised colour ratios:
R/(R+G+B); G/(R+G+B); B/(R+G+B)
Each index has a good relation to the redness/greenness/blueness of strawberry leaves growing in our Strawberry Greenhouse.
A short Python script was written to mask just the leaf pixels and sum the intensities of the red, green and blue pixel values for each leaf. Red, green and blue ratios were then calculated to give numbers independent of illumination intensity. One of the great things about using Python is the ease of programming and the large number of Python library modules freely available. Image analysis was carried out on JPG files using the OpenCV open source computer vision library.
Further work is ongoing to develop image models that reflect the presence of chlorophyll, carotene and anthocyanin pigments more specifically. Ultimately it may be possible to relate changes in pigmentation not only to senescence but also to nutrient levels and disease susceptibility.